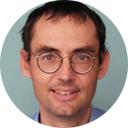
Wulfram Gerstner
After studies of Physics in Tübingen and at the Ludwig-Maximilians-University Munich (Master 1989), Wulfram Gerstner spent a year as a visiting researcher at UC Berkeley. He received his PhD in Theoretical Physics from the Technical University Munich in 1993 with a thesis on associative memory in networks of spiking neurons. After short postdoctoral stays at Brandeis University and the TU Munich, he joined the EPFL in 1996 as Assistant Professor. Promoted to Associate Professor in 2001, he is since 2006 a full professor with double appointment in Computer Science and Life Sciences. Wulfram Gerstner has been invited speaker at numerous international conferences and workshops. He has served on the editorial board of the 'Journal of Computational Neuroscience', and 'Science', as well as other journals. He conducts research in computational neuroscience with special emphasis on models of spiking neurons, spike-timing dependent plasticity, and reward-based learning in spiking neurons.